- Top-of-atmosphere (TOA) reflectance performs a normalization for solar energy. TOA normalizes the pixels values to the 0.0-1.0 range to reduce variability between images. Because the information needed to convert digital numbers (DN) to TOA reflectance values is known, this transformation is very fast and fully automated. The main limitations of TOA reflectance are the remaining effects of Rayleigh scattering (which results in bluish images), aerosol absorption and scattering phenomena (which result in non-uniform, decreased image visibility).
- Rayleigh-only reflectance accounts for the systematic properties of the atmosphere by removing the Rayleigh scattering component (a result of the interaction of sunlight with molecules in the atmosphere) from imagery. Again, the transformation is very fast and fully automated. In cases of very low aerosol loads, Rayleigh-only reflectance produces images that are less blue than TOA reflectance, but the effects of haze variability remain visible.
- Surface reflectance compensates for atmospheric absorption and scattering phenomena on a pixel-by-pixel basis. It approximates what would be measured by a sensor held just above the Earth surface, without any alterations from the atmosphere. Of these three choices, surface reflectance provides the very best visual experience—which is why it’s the method we use.
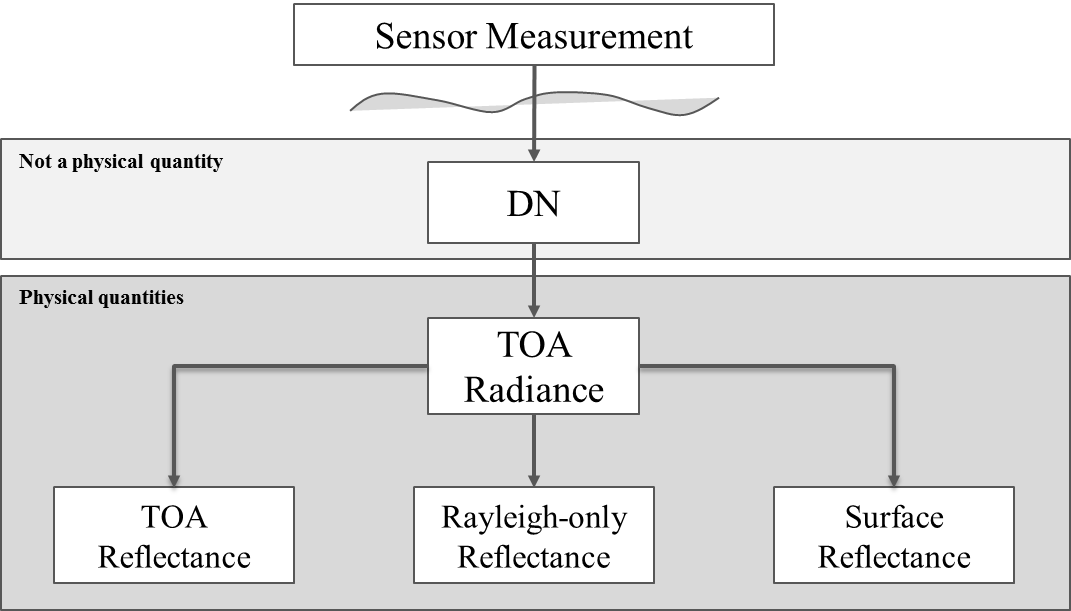
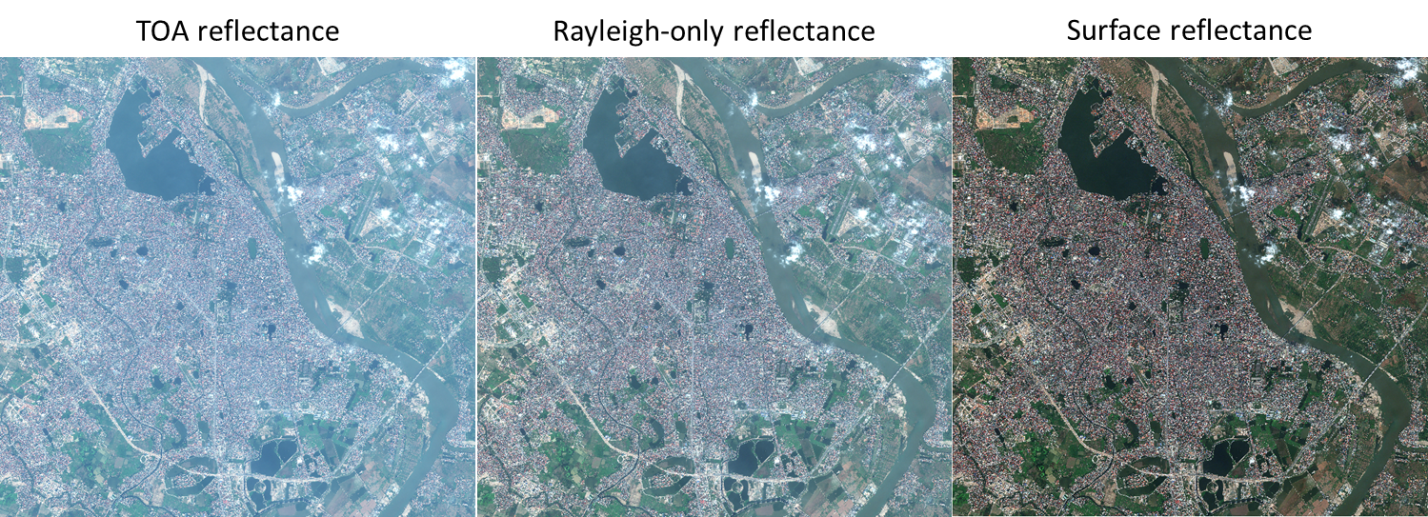
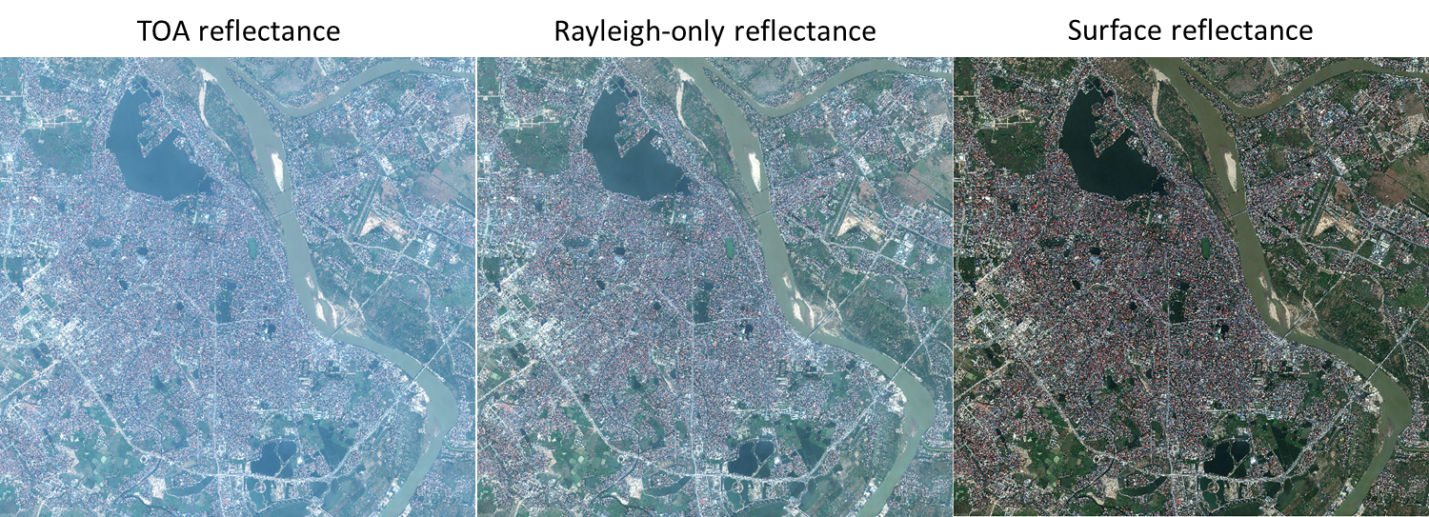
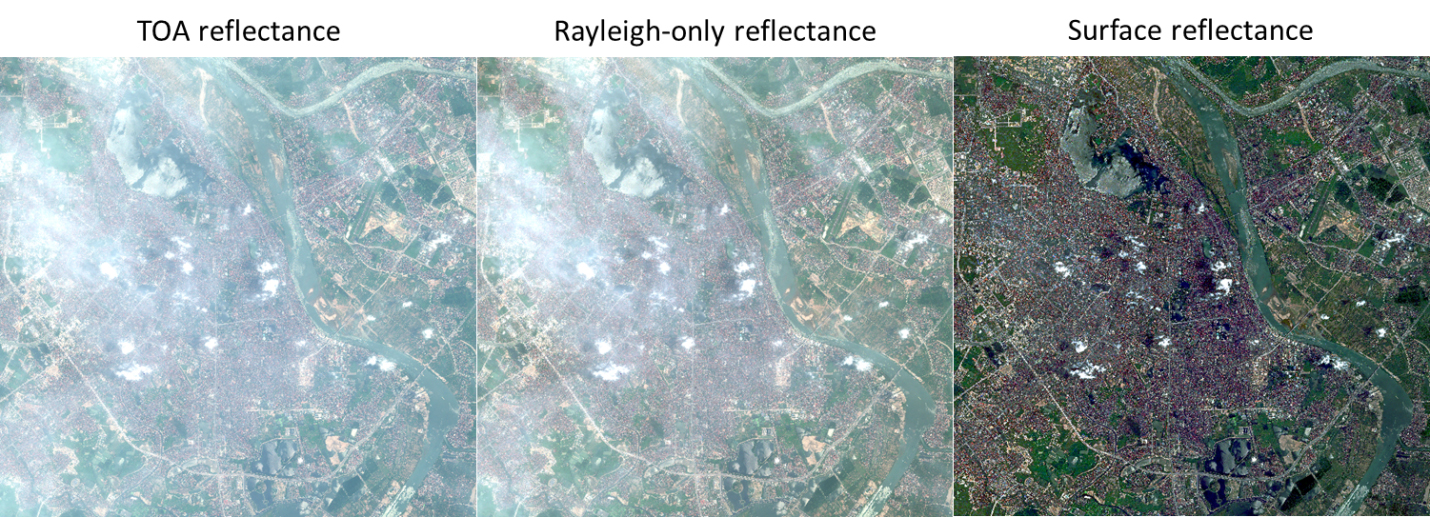
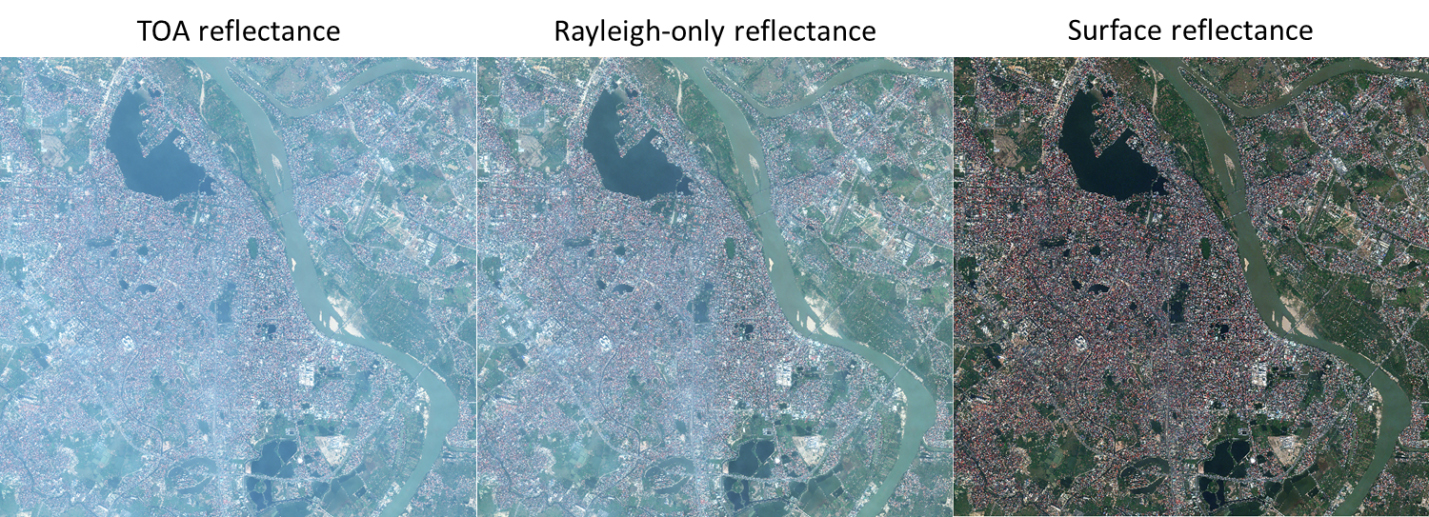
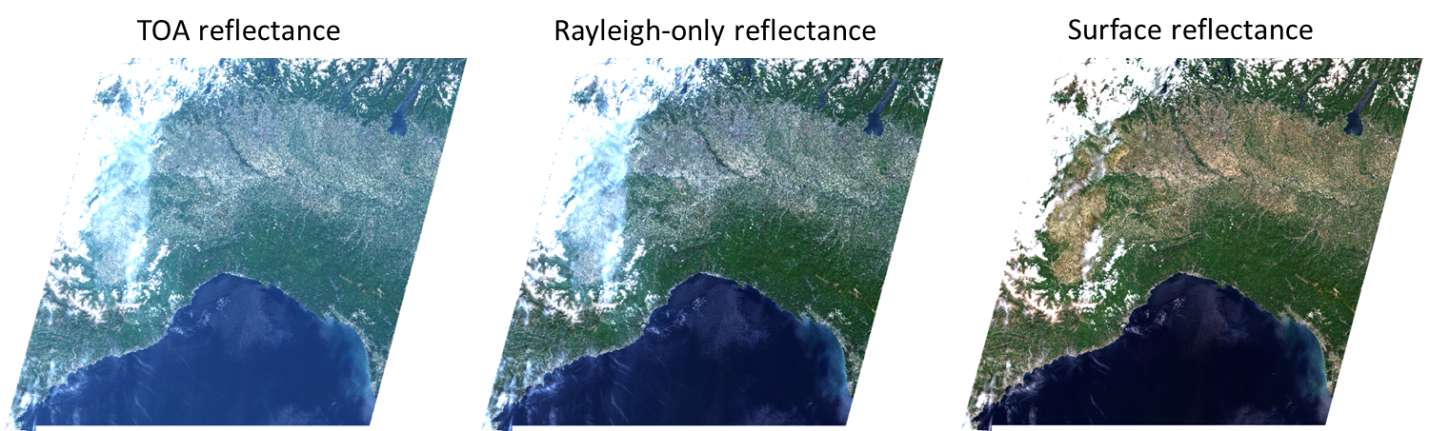
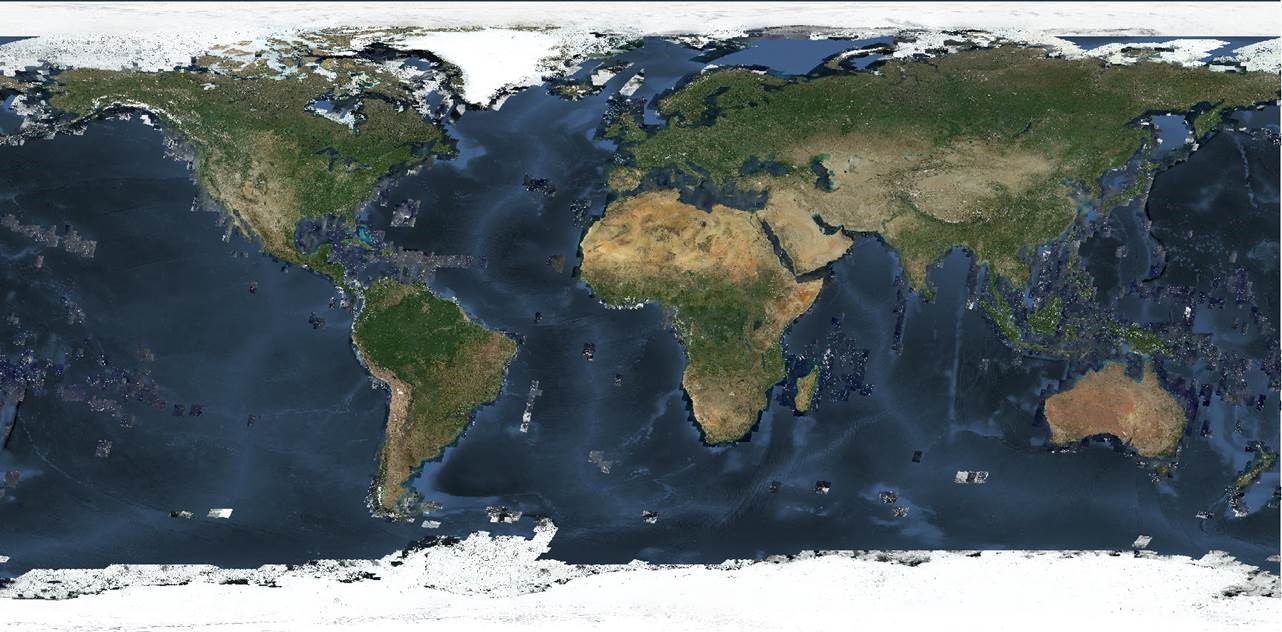